报告题目:基于种群分解的进化多目标和超多目标算法
报告时间:2018年6月22日,星期五,下午3:30-4:30
报告地点:理科楼407
报告摘要:
An effective allocation of search effort is important in multi-objective optimization, particularly in many-objective optimization problems. This paper presents a new adaptive search effort allocation strategy for MOEA/D-M2M, a recent MOEA/D algorithm for challenging Many-Objective Optimization Problems (MaOPs). This proposed method adaptively adjusts the subregions of its subproblems by detecting the importance of different objectives in an adaptive manner. More specifically, it periodically resets the subregion setting based on the distribution of the current solutions in the objective space such that the search effort is not wasted on unpromising regions. The basic idea is that the current population can be regarded as an approximation to the Pareto front (PF) and thus one can implicitly estimate the shape of the PF and such estimation can be used for adjusting the search focus. The performance of proposed algorithm has been verified by comparing it with eight representative and competitive algorithms on a set of degenerated many-objective optimization problems with disconnected and connected PFs. Performances of the proposed algorithm on a number of non-degenerated test instances with connected and disconnected PFs are also studied.
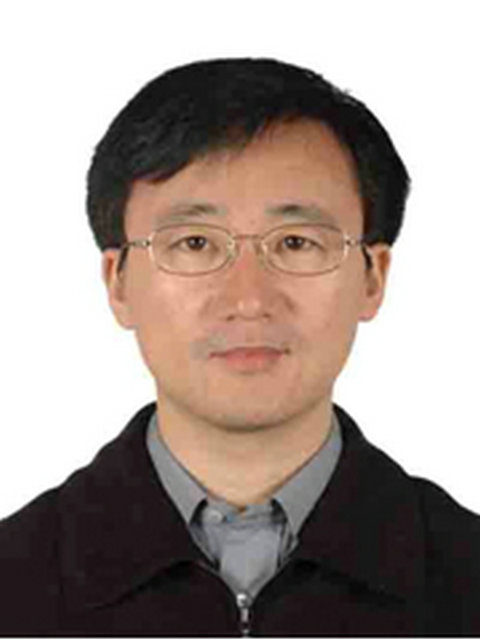
报告人简介:
刘海林博士,广东工业大学应用六合彩网上投注app教授,博士生导师,广东工业大学大数据研究院副院长,广东省现场统计学会副理事长,IEEE的高级会员,IEEE Transaction on Evolutionary Computation副编(Associate Editor)。长期从事进化多目标优化算法及应用研究,近5年来在IEEE Transactions on Evolutionary Computation、IEEE Transactions on Cybernetics、Evolutionary Computation、IEEE Computational Intelligence Magazine等国际期刊发表了30多篇学术论文。