报告题目: Making short-term data predictable by randomly distributed embedding
报告时间:2018年11月9日,星期五,上午9:00
报告地点:六合彩网上投注app
北五楼427
报告人:陈洛南,中国科学院系统生物学重点实验室
报告摘要:
Future state prediction for nonlinear dynamical systems is a challenging task, particularly when only a few time series samples for high-dimensional variables are available from real-world systems. In this work, we propose a model-free framework, named randomly distributed embedding (RDE), to achieve accurate future state prediction based on short-term high-dimensional data. Specifically, from the observed data of high-dimensional variables, the RDE framework randomly generates a sufficient number of low-dimensional “nondelay embeddings” and maps each of them to a “delay embedding,” which is constructed from the data of a to be predicted target variable. Any of these mappings can perform as a low-dimensional weak predictor for future state prediction, and all of such mappings generate a distribution of predicted future states. This distribution actually patches all pieces of association information from various embeddings unbiasedly or biasedly into the whole dynamics of the target variable, which after operated by appropriate estimation strategies, creates a stronger predictor for achieving prediction in a more reliable and robust form. Through applying the RDE framework to data from both representative models and real-world systems, we reveal that a high-dimension feature is no longer an obstacle but a source of information crucial to accurate prediction for short-term data, even under noise deterioration.
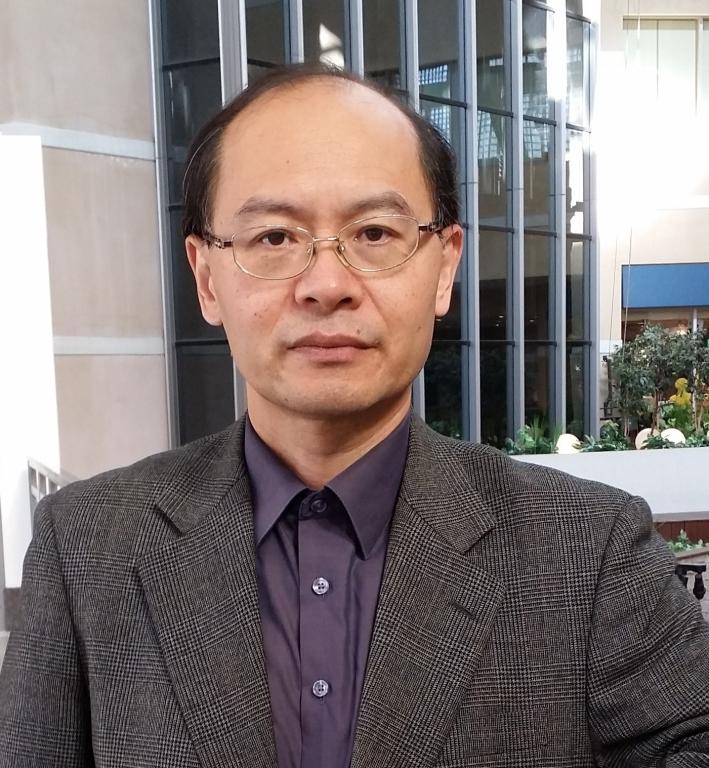
报告人简介:
陈洛南1984年获华中科技大学电气工程学士学位;1988年获日本东北大学系统科学硕士学位;1991年获日本东北大学系统科学博士学位。1997年起任日本大阪产业大学副教授;2000年起任美国加州大学洛杉矶分校(UCLA)访问教授;2002年起任日本大阪产业大学教授;2009年4月起任日本东京大学教授(兼);2009年10月至今任中科院系统生物学重点实验室执行主任,研究员。现任中国运筹学会《计算系统生物学分会》理事长,IEEE-SMC《系统生物学委员会》主席,中日韩国际系统生物学会组织(Trisys)的轮值主席,中国细胞生物学会《功能基因组学与系统生物学分会》副会长,中国药理学会《网络药理学专业委员会》副主任委员,上海市临床生物信息学研究所副所长,国家重点研发计划首席科学家。主要从事计算系统生物学和大数据分析的研究工作。近年来,在《数据驱动的非线性动力学》和《计算系统生物学》等应用数学研究领域发表了300余篇SCI期刊论文及10余部编著书籍 (h-index: 57)。